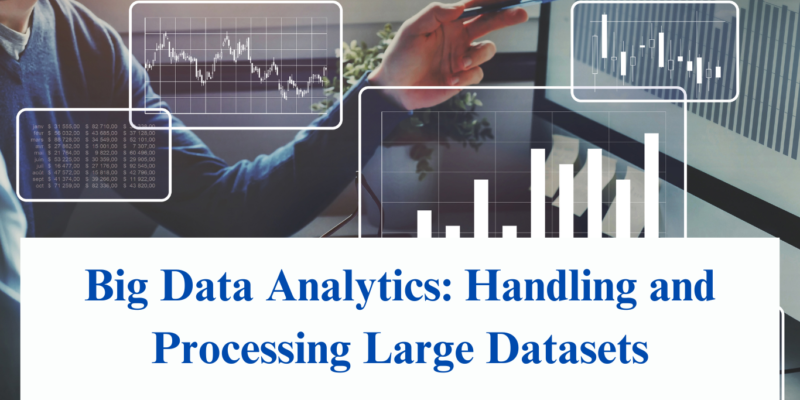
Introduction
In the era of unprecedented data proliferation, the ethical dimensions of data science and the safeguarding of individual privacy have become paramount. As technology advances and organizations leverage data to glean valuable insights, ethical considerations emerge as the cornerstone of responsible data science practices. The intersection of data science, ethics, and privacy raises profound questions about how information is collected, processed, and utilized. The imperative to strike a delicate balance between innovation and the protection of individual rights and privacy underscores the need for responsible data handling. This introduction sets the stage for an in-depth examination of the foundational principles, privacy concerns, and ethical dilemmas shaping the landscape of data science. Join us on a journey into the ethical heart of data handling, where responsible practices serve as the compass guiding the transformative power of data science towards positive and equitable outcomes.
Foundations of Data Ethics
In the intricate landscape of data science, ethical considerations form the bedrock upon which responsible data handling stands. Defined by a commitment to moral principles, data ethics provides the ethical compass that guides practitioners through the multifaceted challenges of data-driven decision-making.
Defining Data Ethics:
At its essence, data ethics encapsulates a set of principles that govern the responsible conduct of data science. These principles extend beyond legal compliance, emphasizing transparency, fairness, and accountability in the collection, processing, and utilization of data. As data science continues to evolve, a clear understanding of these foundational principles becomes imperative to ensure that innovation aligns harmoniously with ethical imperatives.
The Ethical Dilemma:
Amidst the rapid progression of data science, ethical dilemmas emerge as formidable challenges, requiring a delicate balance between technological advancement and ethical considerations. The pursuit of cutting-edge insights must navigate potential pitfalls, including unintended biases, discrimination, and encroachments on privacy. This delicate equilibrium necessitates constant vigilance and an unwavering commitment to ethical practices to navigate the ethical complexities inherent in the dynamic landscape of data science.
In navigating these foundational aspects, practitioners find themselves at the intersection of technological innovation and ethical responsibility. The principles of data ethics provide a moral framework that shapes decision-making processes, emphasizing the need to go beyond mere data compliance. This foundational understanding serves as the ethical cornerstone that guides practitioners in harnessing the transformative power of data science while upholding the principles of fairness, transparency, and accountability.
As organizations and professionals delve deeper into the ethical dimensions of data science, they embark on a journey towards building a responsible data culture. This culture is characterized by a collective commitment to ethical decision-making, fostering trust, and ensuring that the impact of data-driven insights is not only innovative but also aligned with the values and rights of individuals.
Privacy Concerns in Data Science
Privacy concerns loom large in the realm of data science, necessitating a vigilant and ethical approach to safeguard individual rights and personal information. In navigating the complex interplay between data-driven advancements and privacy, it is crucial to understand the intricacies and legal frameworks shaping this dynamic landscape.
The Privacy Paradox:
- The surge in data-driven advancements poses a paradox, balancing the benefits of innovation with the imperative to protect individual privacy.
- As organizations leverage vast datasets for insights, tensions arise between the desire for knowledge and the need to respect personal privacy.
Legal Landscape:
- Governments and regulatory bodies globally acknowledge the significance of safeguarding individual privacy in the digital age.
- Regulatory frameworks like the General Data Protection Regulation (GDPR) in the European Union impose stringent requirements, compelling organizations to adopt responsible data handling practices.
- Compliance with legal standards is integral to ethical data science, ensuring that the rights of individuals are preserved throughout the data lifecycle.
Challenges in Data Collection:
- The complexity of data collection processes presents challenges in obtaining meaningful consent from individuals.
- Communicating the full scope of data collection activities, especially in scenarios involving diverse data types, can be intricate.
- Balancing comprehensive disclosure with avoiding information overload becomes an ongoing ethical challenge.
Striking a Balance:
- Responsible data science involves striking a balance between leveraging data for valuable insights and respecting the privacy rights of individuals.
- Transparency in data handling processes is essential for building trust and empowering individuals to make informed decisions about their data.
Ethics of Data Utilization:
- Ethical data science extends beyond collection to encompass the responsible use and processing of information.
- Practitioners must be mindful of potential privacy infringements and ensure that data utilization aligns with ethical principles.
Understanding and addressing privacy concerns in data science not only comply with legal requirements but also uphold ethical standards. Striking this delicate balance is essential for fostering a data landscape where technological innovation thrives while respecting the fundamental right to privacy.
Informed Consent
Ethical Data Collection:
At the core of responsible data handling lies the concept of informed consent. Ethical data collection necessitates obtaining explicit permission from individuals before gathering their data. This process ensures transparency and empowers individuals with the knowledge of how their information will be utilized. It’s a foundational step in cultivating trust between data practitioners and data subjects.
Challenges in Consent:
Despite the importance of informed consent, challenges arise in obtaining meaningful consent in diverse data scenarios. The complexity of data processing and the potential for unforeseen uses of data make it challenging to communicate the full scope of data collection activities. Striking a balance between comprehensive disclosure and avoiding information overload poses a constant ethical challenge.
Bias and Fairness
Unmasking Bias:
Ethical data handling demands an unwavering commitment to uncovering and addressing biases within datasets and algorithms. Unintended biases can perpetuate discrimination and exacerbate social inequalities. Identifying and understanding these biases is a crucial step in mitigating their impact on decision-making processes.
Algorithmic Fairness:
Achieving fairness in algorithms is a key ethical consideration. Algorithmic fairness involves designing and implementing algorithms that do not discriminate against individuals based on characteristics such as race, gender, or socioeconomic status. It requires continuous monitoring, refinement, and transparency to ensure equitable outcomes in data-driven applications.
The ethical dimensions of informed consent, bias, and fairness underscore the intricate responsibilities inherent in data science practices. The commitment to transparent and fair data collection, coupled with proactive measures to identify and rectify biases, establishes the ethical foundation for fostering trust and ensuring that data science contributes positively to society.
Transparency and Accountability
The Transparency Imperative:
Transparency in data science processes is fundamental to ethical data handling. Organizations must provide clear and understandable explanations of how data is collected, processed, and used. Transparency builds trust and empowers individuals to make informed decisions about their data.
Holding the Line:
Accountability mechanisms are essential in upholding ethical standards in data science. Establishing clear lines of responsibility ensures that individuals and organizations are answerable for the ethical implications of their data practices. Robust accountability frameworks act as safeguards against unethical behavior and promote a culture of responsibility within the data science community.
The twin pillars of transparency and accountability form the bedrock of responsible data handling. As data practitioners strive for openness and take responsibility for the consequences of their actions, ethical considerations become ingrained in the very fabric of data science practices.
Case Studies in Responsible Data Handling
Examining real-world case studies provides insights into the complexities of responsible data handling. Ethical dilemmas may arise when balancing the pursuit of knowledge with the potential impact on individuals and society. Analyzing these cases offers valuable lessons on navigating the ethical challenges inherent in data science.
By delving into case studies, both cautionary tales and success stories, practitioners gain a nuanced understanding of the ethical nuances in data science. Learning from past experiences becomes a guiding principle in shaping future practices, fostering a community of data professionals committed to responsible and ethical data handling. The intricate interplay between transparency, accountability, and real-world applications elucidates the multifaceted nature of ethical considerations in data science.
Striking a balance between innovation and responsibility, these aspects form the ethical fabric that should permeate every stage of the data handling process. As we explore the future of responsible data science, emerging technologies and cultural shifts will further shape the ethical landscape, creating a dynamic space where responsible data handling is not just an obligation but a shared commitment to building a trustworthy data-driven future.
You also Read this Blog:-
Data Analytics: Unlocking Business Potential
Conclusion
In navigating the ethical labyrinth, it becomes evident that the responsible stewardship of data is a collective responsibility. The foundational principles of data ethics, coupled with a commitment to privacy, transparency, and accountability, serve as the pillars that uphold the integrity of data science practices. As we reflect on the complexities and challenges discussed, it is imperative for individuals and organizations to embrace a culture of responsible data handling. This culture extends beyond compliance with legal frameworks; it embodies a genuine commitment to ethical decision-making in every facet of data science. For those aspiring to embark on a journey into the ethical dimensions of data science, enrolling in institutes which provide the Best Online and Offline Data Science Course in Noida, Guwahati, Lucknow, etc. provides not just technical proficiency but a holistic understanding of the ethical considerations that should underscore every data-driven decision. It is through the fusion of knowledge and ethical consciousness that the transformative potential of data science is harnessed responsibly, ensuring a future where innovation coexists harmoniously with ethical imperatives.