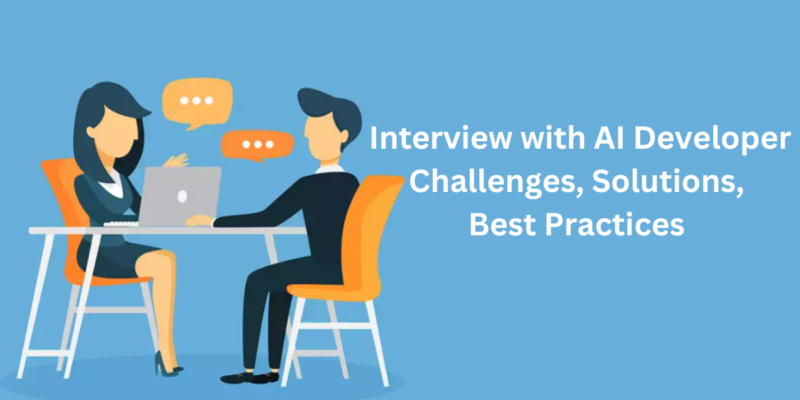
Artificial Intelligence (AI) is rapidly transforming industries, from healthcare to banking, making it one of the most sought-after fields in technology. Today, we sit down with an experienced AI developer from a leading AI development company to explore the intricacies of this evolving industry. This interview sheds light on the key challenges developers face, practical solutions they’ve found, and best practices they’ve honed along the way. Whether you’re a tech enthusiast or a business owner, this article offers invaluable insights into AI’s role in various sectors, including AI in banking, a field that is undergoing a rapid digital transformation.
Introduction to AI Development
The field of AI development involves creating systems capable of performing tasks that typically require human intelligence. These tasks range from understanding natural language to recognizing patterns and making decisions. With AI’s growing role in business operations and customer interactions, the demand for developers is at an all-time high.
But AI development isn’t without its hurdles. From technical challenges to ethical considerations, developers face a wide array of obstacles that require thoughtful solutions and innovative practices.
The Growing Demand for AI Development in Industries
AI is no longer limited to tech giants or research labs. Today, industries such as healthcare, finance, and manufacturing are integrating AI into their processes. One area experiencing a particularly strong demand for AI is the banking sector.
According to our interviewee, an AI expert from a top AI development company, AI in banking is revolutionizing the way institutions manage data, conduct fraud detection, and provide personalized customer services. From virtual assistants to risk management systems, AI is streamlining operations and improving the customer experience.
Key Challenges in AI Development
Despite its transformative potential, AI development is not without significant challenges. According to the developer, the most pressing issues fall into three main categories:
Data Quality and Availability
AI systems rely heavily on vast amounts of high-quality data. In many cases, developers struggle to obtain enough diverse data to train their algorithms effectively. The issue is particularly pronounced in sectors like healthcare and finance, where data privacy laws restrict access to necessary information.
Complexity of Algorithms
Building AI algorithms can be extremely complex. Developers need to strike a balance between creating systems that are sophisticated enough to handle nuanced tasks while also ensuring they remain interpretable and understandable. The increasing complexity can make AI systems difficult to debug and optimize.
Ethical and Security Concerns
Ethical issues, particularly around bias and fairness, pose another significant challenge. AI models are only as unbiased as the data they are trained on, and inadvertent bias can lead to unfair outcomes. Moreover, security concerns, especially in sectors like AI in banking, are crucial. AI systems must be designed to guard against fraud, hacking, and data breaches.
Solutions to Overcome AI Development Challenges
Leveraging Synthetic Data
One solution to the data scarcity issue is the use of synthetic data. Developers are increasingly generating artificial datasets to train AI models, especially in cases where real data is limited or subject to privacy concerns. This technique has proven particularly useful in industries such as healthcare and finance.
Automated Machine Learning (AutoML)
To address the complexity of algorithms, many AI developers are turning to AutoML tools. These tools automate parts of the machine-learning pipeline, making it easier to develop, test, and optimize algorithms. This not only speeds up development but also reduces the chances of human error.
Building Transparent and Explainable AI Models
Transparency is a crucial part of building trust in AI systems. Explainable AI (XAI) techniques are helping developers create models that are easier to interpret. This is especially important in sectors like AI in banking, where customers and regulators need to understand how decisions are being made.
Best Practices for AI Development
When asked about the best practices for AI development, the interviewee emphasized several key points:
Focus on Data Integrity
High-quality, unbiased data is the foundation of any successful AI model. Developers should spend a significant amount of time on data cleaning, preprocessing, and validation. Data quality is especially crucial in AI in banking, where even small errors can have far-reaching financial consequences.
Continuous Learning and Model Updates
- AI models should not be static. Continuous learning is essential for adapting to new data and evolving conditions. In sectors like banking, where market conditions and customer behaviors are always changing, models must be regularly updated to maintain their accuracy and effectiveness.
Cross-Disciplinary Collaboration
AI development isn’t just about coding. It’s essential for developers to collaborate with experts from other fields, such as data science, business strategy, and ethics. This is particularly relevant in areas like AI in banking, where understanding the financial industry is just as important as understanding AI technology.
Security-First Mindset
AI systems, particularly in sensitive industries like banking and healthcare, must prioritize security from the start. Implementing robust security measures, such as encryption and multi-factor authentication, is non-negotiable. AI in banking, for example, requires stringent protocols to protect sensitive customer data and financial transactions.
AI in Banking: A Case Study
AI in banking is one of the most prominent examples of how this technology is transforming traditional industries. From chatbots that handle customer inquiries to advanced systems that detect fraudulent transactions, AI is improving both operational efficiency and customer service.
In the words of the AI developer, “Banking is one of the sectors that have embraced AI the most. The benefits are clear – from faster loan processing times to predictive analytics that help banks offer personalized services to their customers.” AI-powered systems can analyze vast amounts of financial data, predict future trends, and even suggest investment opportunities, all in real-time.
The Future of AI Development
As AI continues to evolve, its applications will only expand. According to the developer, the future of AI lies in greater personalization and automation across industries. In banking, we can expect to see even more sophisticated fraud detection systems, personalized financial advice platforms, and automated customer service tools.
Conclusion
AI development presents a unique set of challenges, but with the right strategies and tools, developers can overcome these hurdles. From improving data quality to implementing transparent and secure models, the insights shared by our AI developer offer a comprehensive roadmap for success.
AI in banking, in particular, stands as a testament to how AI can revolutionize even the most traditional industries, offering faster, smarter, and more secure services. The future is bright for AI, and as developers continue to innovate, we can expect even more groundbreaking applications across sectors.
FAQs
- What is the most significant challenge in AI development today?
The biggest challenge is obtaining high-quality, unbiased data to train AI models. Without sufficient data, it’s challenging to create accurate and reliable systems.
- How is AI impacting the banking sector?
AI in banking is transforming processes like fraud detection, customer service, and loan approval, making them more efficient and personalized.
- What are some best practices for developing AI systems?
Focusing on data quality, maintaining transparency in models, and ensuring security are key best practices in AI development.
- What is Explainable AI (XAI)?
Explainable AI refers to AI models that provide clear, understandable insights into how they make decisions, which is crucial for sectors like banking.
- How can synthetic data help in AI development?
Synthetic data can be used when real data is scarce or restricted, helping developers train their models without violating privacy laws.
- What does the future hold for AI development?
The future of AI includes more personalized services and advanced automation across industries, especially in banking.